Machine learning and data science in materials design: a themed collection
Molecular Systems Design & Engineering Pub Date: 2018-04-25 DOI: 10.1039/C8ME90007H
Abstract
Guest Editors Andrew Ferguson and Johannes Hachmann introduce this themed collection of papers showcasing the latest research leveraging data science and machine learning approaches to guide the understanding and design of hard, soft, and biological materials with tailored properties, function and behaviour.
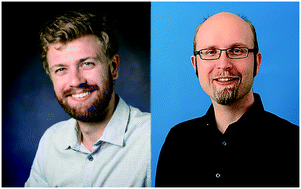
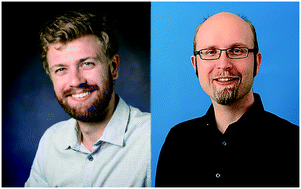
Recommended Literature
- [1] Hybrid lithium-ion capacitors with asymmetric graphene electrodes†
- [2] Back cover
- [3] A general synthesis strategy for one-dimensional Bi2MO6 (M = Mo, W) photocatalysts using an electrospinning method†
- [4] Publications received
- [5] Helical structure in cyclic peptides: effect of N-methyl amides versus esters†
- [6] Back cover
- [7] Interaction of a solid supported liquid-crystalline phospholipidmembrane with physical vapor deposited metal atoms†
- [8] Dual antibacterial activities of a chitosan-modified upconversion photodynamic therapy system against drug-resistant bacteria in deep tissue†
- [9] Mussel and fish scale-inspired underwater superoleophobic kapok membranes for continuous and simultaneous removal of insoluble oils and soluble dyes in water†
- [10] Front cover
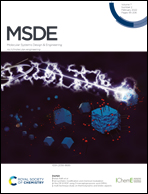
Journal Nameļ¼Molecular Systems Design & Engineering
Research Products
-
CAS no.: 76-83-5
-
CAS no.: 68-94-0
-
3,5-Bis(trifluoromethyl)phenol
CAS no.: 349-58-6
-
CAS no.: 2450-71-7