Machine learning assembly landscapes from particle tracking data†
Soft Matter Pub Date: 2015-08-25 DOI: 10.1039/C5SM01981H
Abstract
Bottom-up self-assembly offers a powerful route for the fabrication of novel structural and functional materials. Rational engineering of self-assembling systems requires understanding of the accessible aggregation states and the structural assembly pathways. In this work, we apply nonlinear machine learning to experimental particle tracking data to infer low-dimensional assembly landscapes mapping the morphology, stability, and assembly pathways of accessible aggregates as a function of experimental conditions. To the best of our knowledge, this represents the first time that collective order parameters and assembly landscapes have been inferred directly from experimental data. We apply this technique to the nonequilibrium self-assembly of metallodielectric Janus colloids in an oscillating electric field, and quantify the impact of field strength, oscillation frequency, and salt concentration on the dominant assembly pathways and terminal aggregates. This combined computational and experimental framework furnishes new understanding of self-assembling systems, and quantitatively informs rational engineering of experimental conditions to drive assembly along desired aggregation pathways.
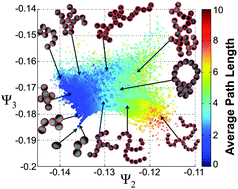
Recommended Literature
- [1] Improvement of the thermoelectric performance of InSe-based alloys doped with Sn†
- [2] General discussion
- [3] Simultaneous determination of dihydroxybenzene isomers using a three-dimensional over-oxidized polypyrrole–reduced graphene oxide composite film electrode prepared by an electrochemical method†
- [4] Formation of β-cyano-ketones through cyanide-promoted ring-opening of cyclic organic carbonates†
- [5] Fine-tuning macrocycle cavity to selectively bind guests in water for near-infrared photothermal conversion†
- [6] Analysis of carbonaceous aerosols: interlaboratory comparison
- [7] Hydrogenation of citral in supercritical CO2 using a heterogeneous Ni(ii) catalyst†
- [8] Distinct global metabolomic profiles of the model organism Caenorhabditis elegans during interactions with Staphylococcus aureus and Salmonella enterica Serovar Typhi†
- [9] Carbazate-modified cross-linked dextran microparticles suppress the progression of osteoarthritis by ROS scavenging†
- [10] Catalytic conversion of chitin as a nitrogen-containing biomass

Journal Name:Soft Matter
Research Products
-
CAS no.: 16817-43-9
-
CAS no.: 178064-02-3
-
CAS no.: 12771-92-5
-
CAS no.: 133556-24-8