Statistical generation of training sets for measuring NO3−, NH4+ and major ions in natural waters using an ion selective electrode array†
Environmental Science: Processes & Impacts Pub Date: 2016-05-03 DOI: 10.1039/C6EM00043F
Abstract
Knowledge of ionic concentrations in natural waters is essential to understand watershed processes. Inorganic nitrogen, in the form of nitrate and ammonium ions, is a key nutrient as well as a participant in redox, acid–base, and photochemical processes of natural waters, leading to spatiotemporal patterns of ion concentrations at scales as small as meters or hours. Current options for measurement in situ are costly, relying primarily on instruments adapted from laboratory methods (e.g., colorimetric, UV absorption); free-standing and inexpensive ISE sensors for NO3− and NH4+ could be attractive alternatives if interferences from other constituents were overcome. Multi-sensor arrays, coupled with appropriate non-linear signal processing, offer promise in this capacity but have not yet successfully achieved signal separation for NO3− and NH4+in situ at naturally occurring levels in unprocessed water samples. A novel signal processor, underpinned by an appropriate sensor array, is proposed that overcomes previous limitations by explicitly integrating basic chemical constraints (e.g., charge balance). This work further presents a rationalized process for the development of such in situ instrumentation for NO3− and NH4+, including a statistical-modeling strategy for instrument design, training/calibration, and validation. Statistical analysis reveals that historical concentrations of major ionic constituents in natural waters across New England strongly covary and are multi-modal. This informs the design of a statistically appropriate training set, suggesting that the strong covariance of constituents across environmental samples can be exploited through appropriate signal processing mechanisms to further improve estimates of minor constituents. Two artificial neural network architectures, one expanded to incorporate knowledge of basic chemical constraints, were tested to process outputs of a multi-sensor array, trained using datasets of varying degrees of statistical representativeness to natural water samples. The accuracy of ANN results improves monotonically with the statistical representativeness of the training set (error decreases by ∼5×), while the expanded neural network architecture contributes a further factor of 2–3.5 decrease in error when trained with the most representative sample set. Results using the most statistically accurate set of training samples (which retain environmentally relevant ion concentrations but avoid the potential interference of humic acids) demonstrated accurate, unbiased quantification of nitrate and ammonium at natural environmental levels (±20% down to <10 μM), as well as the major ions Na+, K+, Ca2+, Mg2+, Cl−, and SO42−, in unprocessed samples. These results show promise for the development of new in situ instrumentation for the support of scientific field work.
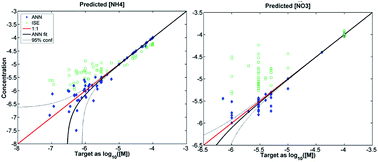
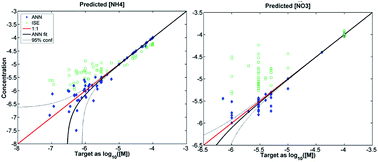
Recommended Literature
- [1] New-generation integrated devices based on dye-sensitized and perovskite solar cells
- [2] Index of subjects
- [3] Comparison between direct current and radiofrequency glow discharge mass spectrometry for the analysis of oxide-based samples
- [4] Insertion of pyridine into an iron–silicon bond: structure of the product Cp*(CO)Fe{η3(C,C,C)-C5H5NSiMe2NPh2}†
- [5] Iridium(i)-catalyzed vinylic C–H borylation of 1-cycloalkenecarboxylates with bis(pinacolato)diboron†
- [6] Light-emitting diode (LED)-directed green synthesis of silver nanoparticles and evaluation of their multifaceted clinical and biological activities
- [7] Results report of apatite fission-track analysis by LA-ICP-MS and its comparison with the conventional external detector method of dating†
- [8] Response of CPO-27-Ni towards CO, N2 and C2H4
- [9] A cobalt phosphide catalyst for the hydrogenation of nitriles†
- [10] Reconfigurable OR and XOR logic gates based on dual responsive on–off–on micromotors†
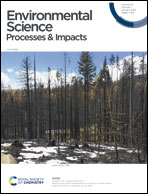
Journal Name:Environmental Science: Processes & Impacts
Research Products
-
CAS no.: 137361-05-8