Will it crystallise? Predicting crystallinity of molecular materials†
CrystEngComm Pub Date: 2014-11-04 DOI: 10.1039/C4CE01912A
Abstract
Predicting and controlling crystallinity of molecular materials has applications in a crystal engineering context, as well as process control and formulation in the pharmaceutical industry. Here, we present a machine learning approach to this problem which uses a large input training set which is classified on a single measurable outcome: does a substance have a reasonable probability of forming good quality crystals. While the related problem of crystal structure prediction requires reliable calculation of three dimensional molecular conformations, the method employed here for predicting crystallisation propensity uses only “two dimensional” information consisting of atom types and connectivity. We show that an error rate lower than 10% can be achieved against unseen test data. The predictive model was also tested in a blind screen of a set of compounds which do not have crystal structures reported in the literature, and we found it to have a 79% classification accuracy. Analysis of the most significant descriptors used in the classification shows that the number of rotatable bonds and a molecular connectivity index are key in determining crystallisation propensity and using these two measures alone can give 80% accurate classification of unseen test data.
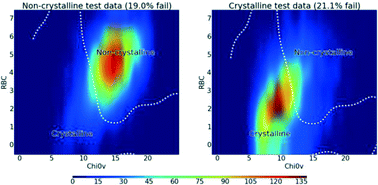
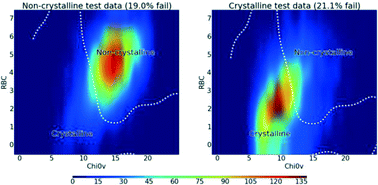
Recommended Literature
- [1] A distorted trigonal bipyramidal co-ordination of cobalt in tris-(o-diphenylphosphinophenyl)phosphinochlorocobalt(II) tetraphenylborate
- [2] Front cover
- [3] Contents list
- [4] Creating SERS hot spots on ultralong single-crystal β-AgVO3 microribbons†
- [5] A natural hyperoside based novel light-up fluorescent probe with AIE and ESIPT characteristics for on-site and long-term imaging of β-galactosidase in living cells†
- [6] Diagnosing the plasma formed during acoustic cavitation in [BEPip][NTf2] ionic liquid
- [7] Perphenazine–fumaric acid salts with improved solubility: preparation, physico-chemical characterization and in vitro dissolution†
- [8] Supramolecular structures formed by 2-aminopyridine derivatives. Part I. Hydrogen-bonding networks via N–H⋯N interactions and the conformational polymorphism of N,N′-bis(2-pyridyl)aryldiamines
- [9] Production of polyetheretherketone in ionic liquid media
- [10] Thermal expansion of coals and carbonised coals