A Bayesian graph convolutional network for reliable prediction of molecular properties with uncertainty quantification†
Chemical Science Pub Date: 2019-07-22 DOI: 10.1039/C9SC01992H
Abstract
Deep neural networks have been increasingly used in various chemical fields. In the nature of a data-driven approach, their performance strongly depends on data used in training. Therefore, models developed in data-deficient situations can cause highly uncertain predictions, leading to vulnerable decision making. Here, we show that Bayesian inference enables more reliable prediction with quantitative uncertainty analysis. Decomposition of the predictive uncertainty into model- and data-driven uncertainties allows us to elucidate the source of errors for further improvements. For molecular applications, we devised a Bayesian graph convolutional network (GCN) and evaluated its performance for molecular property predictions. Our study on the classification problem of bio-activity and toxicity shows that the confidence of prediction can be quantified in terms of the predictive uncertainty, leading to more accurate virtual screening of drug candidates than standard GCNs. The result of log P prediction illustrates that data noise affects the data-driven uncertainty more significantly than the model-driven one. Based on this finding, we could identify artefacts that arose from quantum mechanical calculations in the Harvard Clean Energy Project dataset. Consequently, the Bayesian GCN is critical for molecular applications under data-deficient conditions.
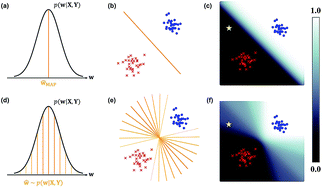
Recommended Literature
- [1] An ultrasensitive detection of 17β-estradiol using a gold nanoparticle-based fluorescence immunoassay†
- [2] Kinetic isotope effects in proton-transfer reactions
- [3] Enhanced structural and magnetic properties of fcc colloidal crystals of cobalt nanoparticles
- [4] Chitin/egg shell membrane@Fe3O4 nanocomposite hydrogel for efficient removal of Pb2+ from aqueous solution†
- [5] Non-emissive plastic colour filters for fluorescence detection
- [6] Synthesis of 3C-alkylated active methylene substituted 2H-indazole derivatives via sequential ring opening of donor–acceptor cyclopropanes and reductive cyclization reaction†
- [7] Tetramethylpyrazine: an electrolyte additive for high capacity and energy efficiency lithium–oxygen batteries†
- [8] Quantitative method for determining the concentration of mercury(II) sulphide in soils and sediments
- [9] Functionalizing the pore wall of chiral porous metal–organic frameworks by distinct –H, –OH, –NH2, –NO2, –COOH shutters showing selective adsorption of CO2, tunable photoluminescence, and direct white-light emission†
- [10] Trace level analysis of polycyclic aromatic hydrocarbons in surface waters by solid phase extraction (SPE) and gas chromatography-ion trap mass spectrometry (GC-ITMS)

Journal Name:Chemical Science
Research Products
-
CAS no.: 13370-08-6
-
CAS no.: 16881-33-7
-
CAS no.: 177966-61-9
-
CAS no.: 133556-24-8