Benchmarking protein structure predictors to assist machine learning-guided peptide discovery†
Digital Discovery Pub Date: 2023-06-02 DOI: 10.1039/D3DD00045A
Abstract
Machine learning models provide an informed and efficient strategy to create novel peptide and protein sequences with the desired profiles. Nevertheless, they are primarily trained on sequences where the tridimensional structures of peptides and proteins are often overlooked. We need a fast and reliable approach to estimate the structural diversity of medium-large training sets before building models. This study benchmarked four protein structure prediction methods (Jpred4, PEP2D, PSIPRED, AlphaFold2) using 261 curated and experimentally known structures from the PDBe database. We applied our best predictor to map the structural landscape of GRAMPA, the giant and vastly uncharted repository of 5980 antimicrobial peptides. The dataset was predominantly made of loose helices (65.1%), followed by random coils (17.8%), and β-stranded and mixed structures accounted for the rest.
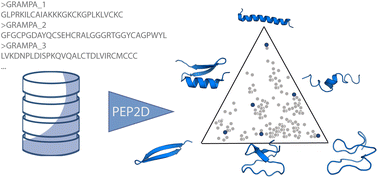
Recommended Literature
- [1] Morphology modulation and application of Au(i)–thiolate nanostructures†
- [2] Contents pages
- [3] Determination of quinolone antibiotics in honey by pH-induced natural deep eutectic solvent combined with vortex-assisted dispersive liquid–liquid microextraction†
- [4] Production of butanol from lignocellulosic biomass: recent advances, challenges, and prospects
- [5] Back cover
- [6] Selective catalytic etherification of glycerol formal and solketal with dialkyl carbonates and K2CO3†
- [7] Synthesis and characterization of 1,3,4,6-tetraarylpyrrolo[3,2-b]-pyrrole-2,5-dione (isoDPP)-based donor–acceptor polymers with low band gap†
- [8] Kinetic and structural understanding of bulk and supported vanadium-based catalysts for furfural oxidation to maleic anhydride†
- [9] Front cover
- [10] Fluorescent pH sensors with negligible sensitivity to ionic strength
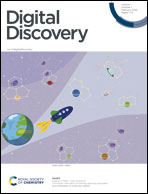