Application of deep learning to support peak picking during non-target high resolution mass spectrometry workflows in environmental research†
Environmental Science: Advances Pub Date: 2023-04-19 DOI: 10.1039/D3VA00005B
Abstract
With the advent of high-resolution mass spectrometry (HRMS), untargeted analytical approaches have become increasingly important across many different disciplines including environmental fields. However, analysing mass spectra produced by HRMS can be challenging due to the sensitivity of low abundance analytes, the complexity of sample matrices and the volume of data produced. This is further compounded by the challenge of using pre-processing algorithms to reliably extract useful information from the mass spectra whilst removing experimental artefacts and noise. It is essential that we investigate innovative technology to overcome these challenges and improve analysis in this data-rich area. The application of artificial intelligence to support data analysis in HRMS has a strong potential to improve current approaches and maximise the value of generated data. In this work, we investigated the application of a deep learning approach to classify MS peaks shortlisted by pre-processing workflows. The objective was to classify extracted ROIs into one of three classes to sort feature lists for downstream data interpretation. We developed and compared several convolutional neural networks (CNN) for peak classification using the Python library Keras. The optimized CNN demonstrated an overall accuracy of 85.5%, a sensitivity of 98.8% and selectively of 97.8%. The CNN approach rapidly and accurately classified peaks, reducing time and costs associated with manual curation of shortlisted features after peak picking. This will further support interpretation and understanding from this discovery-driven area of analytical science.
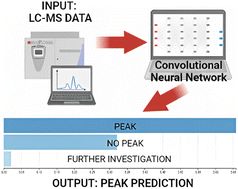
Recommended Literature
- [1] Real-time monitoring of epithelial barrier function by impedance spectroscopy in a microfluidic platform†
- [2] Decoration of boron nanoparticles on a graphene sheet for ammonia production from nitrate†
- [3] Influence of Li2O2 morphology on oxygen reduction and evolution kinetics in Li–O2 batteries†
- [4] Front cover
- [5] Inside front cover
- [6] Front cover
- [7] Influence of film-thickness on the ozone detection of perovskite La0.8Pb0.1Ca0.1Fe1−xCoxO3 based sensors
- [8] Oxyhemoglobin nano-recruiter preparation and its application in biomimetic red blood cells to relieve tumor hypoxia and enhance photodynamic therapy activity†
- [9] Photoinduced electron transfer in a supramolecular triad produced by porphyrin anion-induced electron transfer from tetrathiafulvalene calix[4]pyrrole to Li+@C60†
- [10] Synthetic-biology-based discovery of a fungal macrolide from Macrophomina phaseolina†
Journal Name:Environmental Science: Advances
Research Products
-
CAS no.: 10424-09-6
-
CAS no.: 11016-71-0
-
CAS no.: 12025-32-0