Machine learning-inspired battery material innovation
Energy Advances Pub Date: 2023-02-22 DOI: 10.1039/D3YA00040K
Abstract
Machine learning (ML) techniques have been a powerful tool responsible for many new discoveries in materials science in recent years. In the field of energy storage materials, particularly battery materials, ML techniques have been widely utilized to predict and discover materials’ properties. In this review, we first discuss the key properties of the most common electrode and electrolyte materials. We then summarize recent progress in battery material advancement using ML techniques, through the three main strategies of direct property predictions, machine learning potentials, and inverse design. The major challenges, advantages and limitations of these techniques are also discussed. Finally, we conclude this review with a perspective on sustainable battery development using ML.
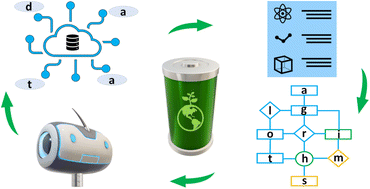
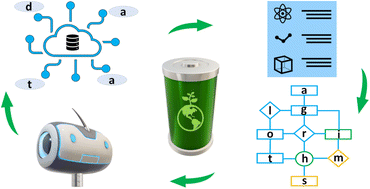
Recommended Literature
- [1] Contents list
- [2] Acrylamide risk in food products: The shortbread case study
- [3] Manipulation of biological samples using micro and nano techniques
- [4] A comprehensive review on the fabrication, modification and applications of Na3V2(PO4)2F3 cathodes
- [5] Plant natural products with leishmanicidal activity
- [6] Efficient nitrogen-13 radiochemistry catalyzed by a highly stable immobilized biocatalyst†
- [7] Strategically integrating quantum dots into organic and perovskite solar cells
- [8] A Langmuir-like desorption model for reflecting the inhomogeneous pore structure of coal and its experimental verification
- [9] Crystal structure, electronic properties and cytotoxic activity of palladium chloride complexes with monosubstituted pyridines†
- [10] Inside front cover
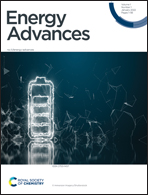
Journal Name:Energy Advances
Research Products
-
CAS no.: 174064-00-7
-
CAS no.: 170643-02-4