A machine learning-based framework to design capillary-driven networks
Lab on a Chip Pub Date: 2022-11-08 DOI: 10.1039/D2LC00843B
Abstract
We present a novel approach for the design of capillary-driven microfluidic networks using a machine learning genetic algorithm (ML-GA). This strategy relies on a user-friendly 1D numerical tool specifically developed to generate the necessary data to train the ML-GA. This 1D model was validated using analytical results issued from a Y-shaped capillary network and experimental data. For a given microfluidic network, we defined the objective of the ML-GA to obtain the set of geometric parameters that produces the closest matching results against two prescribed curves of delivered volume against time. We performed more than 20 generations of 10 000 simulations to train the ML-GA and achieved the optimal solution of the inverse design problem. The optimisation took less than 6 hours, and the results were successfully validated using experimental data. This work establishes the utility of the presented method for the fast and reliable design of complex capillary-driven devices, enabling users to optimise their designs via an easy-to-use 1D numerical tool and machine learning technique.
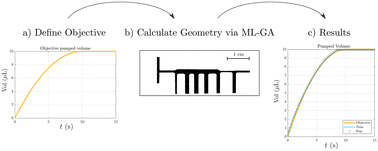
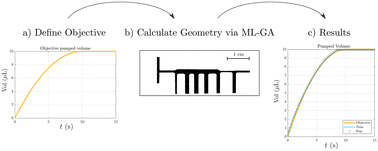
Recommended Literature
- [1] Syntheses and post-functionalization of tri-substituted polyalkoxohexavanadates containing tris(alkoxo) ligands†
- [2] Inside back cover
- [3] Zinc-assisted mechanochemical coating of a reduced graphene oxide thin layer on silicon microparticles to achieve efficient lithium-ion battery anodes†
- [4] Photoelectrochemical production of useful fuels from carbon dioxide on a polypyrrole-coated p-ZnTe photocathode under visible light irradiation†
- [5] Carbene catalyzed umpolung of α,β-enals: a reactivity study of diamino dienols vs. azolium enolates, and the characterization of advanced reaction intermediates†
- [6] Genoprotective effects of gallic acid against cisplatin induced genotoxicity in bone marrow cells of mice
- [7] The structural fate of lipid nanoparticles in the extracellular matrix†
- [8] General and physical chemistry
- [9] Front cover
- [10] Xeno nucleic acids (XNAs) having non-ribose scaffolds with unique supramolecular properties
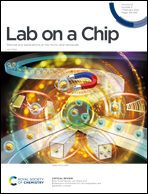
Journal Name:Lab on a Chip
Research Products
-
CAS no.: 104-50-7
-
CAS no.: 515-94-6
-
4-(Trifluoromethyl)benzaldehyde
CAS no.: 455-19-6
-
CAS no.: 2450-71-7
-
CAS no.: 83-38-5
-
Methyl 4-(bromomethyl)benzoate
CAS no.: 2417-72-3
-
2-(Morpholinothio)benzothiazole
CAS no.: 102-77-2