Scalable graph neural network for NMR chemical shift prediction
Physical Chemistry Chemical Physics Pub Date: 2022-10-17 DOI: 10.1039/D2CP04542G
Abstract
Graph neural networks (GNNs) have been proven effective in the fast and accurate prediction of nuclear magnetic resonance (NMR) chemical shifts of a molecule. Existing methods, despite their effectiveness, suffer from high space complexity and are therefore limited to relatively small molecules. In this work, we propose a scalable GNN for NMR chemical shift prediction. To reduce the space complexity, we sparsify the graph representation of a molecule by regarding only heavy atoms as nodes and their chemical bonds as edges. To better learn from the sparsified graph representation, we improve the message passing and readout functions in the GNN. For the message passing function, we adapt the attention mechanism and residual connection to better capture local information around each node. For the readout function, we use both node-level and graph-level embeddings as the local and global information to better predict node-level chemical shifts. Through the experimental investigation using 13C and 1H NMR datasets, we demonstrate that the proposed method yields higher prediction accuracy and is more scalable to large molecules having many heavy atoms.
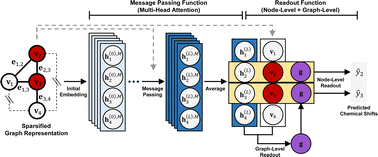
Recommended Literature
- [1] The kinetics of phase transitions in underpotentially deposited Cu adlayers on Au(111)
- [2] Graphene quantum dots/graphene fiber nanochannels for osmotic power generation†
- [3] Bio-based thermoset composites from epoxidised linseed oil and expanded starch†
- [4] Exploring the mechanical properties of nanometer-thick elastic films through micro-drop impinging on large-area suspended graphene†
- [5] Cobalt nanoparticles encapsulated in nitrogen-doped carbon as a bifunctional catalyst for water electrolysis†
- [6] Dissolution behavior of precious metals and selective palladium leaching from spent automotive catalysts by trihalide ionic liquids†
- [7] Multifunctional Gd-based mesoporous silica nanotheranostic for anticancer drug delivery†
- [8] New member of council
- [9] How metallylenes activate small molecules†
- [10] Solvent dependent competition between fluorescence resonance energy transfer and through bond energy transfer in rhodamine appended hexaphenylbenzene derivatives for sensing of Hg2+ ions†

Journal Name:Physical Chemistry Chemical Physics
Research Products
-
CAS no.: 108373-05-3
-
CAS no.: 10383-90-1
-
CAS no.: 13446-44-1