Predicting aggregate morphology of sequence-defined macromolecules with recurrent neural networks†
Soft Matter Pub Date: 2022-06-15 DOI: 10.1039/D2SM00452F
Abstract
Self-assembly of dilute sequence-defined macromolecules is a complex phenomenon in which the local arrangement of chemical moieties can lead to the formation of long-range structure. The dependence of this structure on the sequence necessarily implies that a mapping between the two exists, yet it has been difficult to model so far. Predicting the aggregation behavior of these macromolecules is challenging due to the lack of effective order parameters, a vast design space, inherent variability, and high computational costs associated with currently available simulation techniques. Here, we accurately predict the morphology of aggregates self-assembled from sequence-defined macromolecules using supervised machine learning. We find that regression models with implicit representation learning perform significantly better than those based on engineered features such as k-mer counting, and a recurrent-neural-network-based regressor performs the best out of nine model architectures we tested. Furthermore, we demonstrate the high-throughput screening of monomer sequences using the regression model to identify candidates for self-assembly into selected morphologies. Our strategy is shown to successfully identify multiple suitable sequences in every test we performed, so we hope the insights gained here can be extended to other increasingly complex design scenarios in the future, such as the design of sequences under polydispersity and at varying environmental conditions.
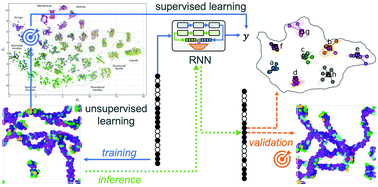
Recommended Literature
- [1] Low-molecular-mass iron in healthy blood plasma is not predominately ferric citrate†
- [2] Synthesis, structural characterization and photoluminescence property of four di(mono)acylhydrazidate-coordinated Cd2+ and Zn2+ compounds†
- [3] Back cover
- [4] Correction: Breath figure in non-aqueous vapor
- [5] Visible to infrared plasmonic absorption from silver nanostructures enabled by microreactor-assisted solution deposition
- [6] Entwined dimer formation from self-complementary bis-acridiniums†
- [7] Complete 1H and 13C NMR assignment and 31P NMR determination of pentacyclic triterpenic acids†
- [8] Controllable synthesis of brookite/anatase/rutile TiO2 nanocomposites and single-crystalline rutilenanorods array†
- [9] Back matter
- [10] The specific vibrational modes of GTP in solution and bound to Ras: a detailed theoretical analysis by QM/MM simulations†

Journal Name:Soft Matter
Research Products
-
CAS no.: 16881-33-7
-
CAS no.: 140632-19-5