Hybrid classical/machine-learning force fields for the accurate description of molecular condensed-phase systems†
Chemical Science Pub Date: 2023-10-31 DOI: 10.1039/D3SC04317G
Abstract
Electronic structure methods offer in principle accurate predictions of molecular properties, however, their applicability is limited by computational costs. Empirical methods are cheaper, but come with inherent approximations and are dependent on the quality and quantity of training data. The rise of machine learning (ML) force fields (FFs) exacerbates limitations related to training data even further, especially for condensed-phase systems for which the generation of large and high-quality training datasets is difficult. Here, we propose a hybrid ML/classical FF model that is parametrized exclusively on high-quality ab initio data of dimers and monomers in vacuum but is transferable to condensed-phase systems. The proposed hybrid model combines our previous ML-parametrized classical model with ML corrections for situations where classical approximations break down, thus combining the robustness and efficiency of classical FFs with the flexibility of ML. Extensive validation on benchmarking datasets and experimental condensed-phase data, including organic liquids and small-molecule crystal structures, showcases how the proposed approach may promote FF development and unlock the full potential of classical FFs.
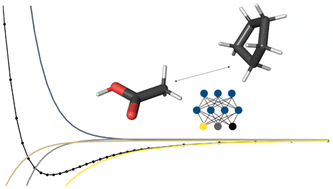
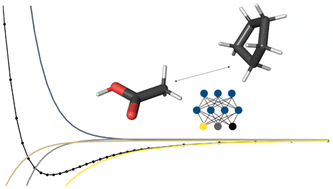
Recommended Literature
- [1] Characterization of optical manipulation using microlens arrays depending on the materials and sizes in organic photovoltaics†
- [2] The interaction of an amorphous metal–organic cage-based solid (aMOC) with miRNA/DNA and its application on a quartz crystal microbalance (QCM) sensor†
- [3] Adsorption and binding of capping molecules for highly luminescent CdSenanocrystals – DFT simulation studies†
- [4] Accessing slow diffusion in solids by employing metadynamics simulation†
- [5] Structure–property relationships based on Hammett constants in cyclometalated iridium(iii) complexes: their application to the design of a fluorine-free FIrPic-like emitter†
- [6] Milk-blended butter. King's Bench Division
- [7] Back cover
- [8] Oxalate promoted iron dissolution of hematite via proton coupled electron transfer†
- [9] Olefin metathesis in air using latent ruthenium catalysts: imidazole substituted amphiphilic hydrogenated ROMP polymers providing nano-sized reaction spaces in water†
- [10] Unraveling multiple binding modes of acridine orange to DNA using a multispectroscopic approach