Machine learning-based q-RASPR predictions of detonation heat for nitrogen-containing compounds†
Materials Advances Pub Date: 2023-10-24 DOI: 10.1039/D3MA00535F
Abstract
The quantitative Read-Across Structure–Property Relationship (q-RASPR) is a novel method for the property predictions derived from the integrated concept of both similarity-based predictions (i.e., Read-Across or RA) and statistical modelling-based predictions (i.e., Quantitative Structure–Property Relationship or QSPR). The main performance index of ammunition used in air-to-air and underwater weapons is the detonation heat energy. In the present work, we have applied the q-RASPR modeling approach and various Machine Learning (ML) algorithms to predict the detonation heat (an intrinsic property) of different N-containing compounds. The data set was collected from the literature, curated, and further divided into training and test sets using the Euclidean distance-based algorithm. The feature selection was done on the basis of internal validation metrics of Genetic Algorithm (GA) models. A Multiple Linear Regression (MLR) QSPR model with 6 descriptors was selected, and the model features were used to calculate the similarity and error-based RASPR descriptors. The RASPR descriptor matrix was then merged with the features of the QSPR model. A grid search was performed for the selection of a combination of descriptors which were then subjected to Partial Least Squares (PLS) regression to obviate the inter-correlation among the descriptors. We have also employed various ML algorithms by optimizing the hyperparameters based on a cross-validation approach and compared the final test set prediction results. The PLS q-RASPR model was selected to be the best model based on the external validation metrics and it also shows enhanced prediction quality using 2D-descriptors compared to the previous model reported with 3D-descriptors. The developed model can be used for the detection of the detonation heat of compounds containing nitrogen with an effective performance.
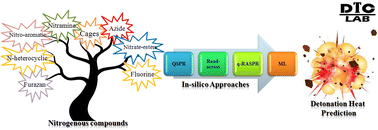
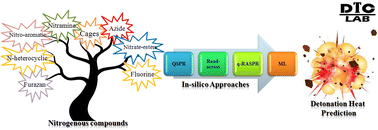
Recommended Literature
- [1] Dendrite inhibited and dead lithium activated dual-function additive for lithium metal batteries†
- [2] Synthesis of curtain-like crumpled boehmite and γ-alumina nanosheets†
- [3] Electrophoretic deposition of TiO2 nanorods for low-temperature dye-sensitized solar cells
- [4] Selective and general exhaustive cross-coupling of di-chloroarenes with a deficit of nucleophiles mediated by a Pd–NHC complex†
- [5] Inside front cover
- [6] S 77. α-Decay systematics
- [7] Wash-free non-spectroscopic optical immunoassay by controlling retroreflective microparticle movement in a microfluidic chip†
- [8] Contents list
- [9] Back matter
- [10] Investigating perceptions of the structure and development of scientific knowledge in the context of a transformed organic chemistry lecture course

Journal Name:Materials Advances
Research Products
-
CAS no.: 14674-74-9
-
CAS no.: 101713-87-5