Machine learning method aided discovery of the fourth-generation EGFR inhibitors†‡
New Journal of Chemistry Pub Date: 2023-11-06 DOI: 10.1039/D3NJ03204C
Abstract
Epidermal growth factor receptor (EGFR) mutations are identified as driver mutations in non-small cell lung cancer (NSCLC), but drug resistance is the key issue. With third-generation EGFR inhibitors having been used for treatment for a longer period of time, designing potent EGFR inhibitors that overcome drug resistance is a crying need, in which the fourth-generation EGFR inhibitors are very promising. In this work, classification models and regression models were constructed to assist in the discovery of the fourth-generation EGFR inhibitors. By using a combination of eight machine-learning (ML) approaches and three strategies, presently, 24 classification models for distinguishing whether it is an EGFR inhibitor were constructed. Among these models, the SVM model exhibits the best performance, with accuracy (ACC), ROC area under the curve (ROC) and Matthews correlation coefficient (MCC) values at 95.5%, 92.4% and 84.7% for the external validation set, respectively. In addition, after using recursive feature elimination (RFE), an efficient approach for feature filtering, to screen the high-dimensional and massive molecular descriptors, 10 regression models including 5 single models and 5 combined models for estimating the inhibitory potency were built. The combined model RF-RFE-SVM shows the best prediction capacity with Rtest2 = 0.93. With the attempt to analyze the contribution of features to models, the SHapley Additive explanation (SHAP) method was also adopted when interpreting the obtained models. Thereafter, based on the feature importance, compounds were selected to construct pharmacophore models and for molecular docking, for further studying the key pharmacodynamic characteristics (hydrogen bonding acceptor for an sp2 hybridized oxygen atom and an alkyl-type hydrophobic group) as well as the interactions (hydrogen bonding interactions and hydrophobic interactions) between the inhibitors and the EGFR protein, respectively. Collectively, the findings support the discovery of lead compounds of the fourth-generation EGFR inhibitors, highlighting a strong potential of machine learning in drug discovery.
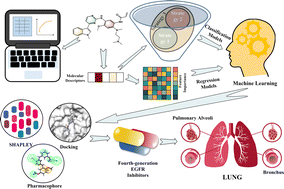
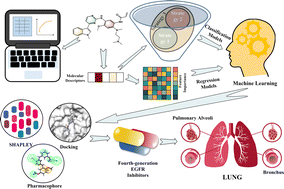
Recommended Literature
- [1] Simultaneous extraction of four classes of antibiotics in soil, manure and sewage sludge and analysis by liquid chromatography-tandem mass spectrometry with the isotope-labelled internal standard method
- [2] Pyridine imines as ligands in luminescent iridium complexes†
- [3] From helicate to infinite coordination polymer: crystal and molecular structures of silver(I) complexes of readily prepared di-Schiff bases
- [4] Salt modified starch: sustainable, recyclable plastics
- [5] Ligand-modified synthesis of shape-controllable and highly luminescent CsPbBr3 perovskite nanocrystals under ambient conditions†
- [6] Competitive or sequential reaction of an electrophilic terminal phosphinidene metal(0) complex with allyl halides? [2+1]-cycloaddition vs. C–X bond insertion†
- [7] Structural and chemical interplay between nano-active and encapsulation materials in a core–shell SnO2@MXene lithium ion anode system†
- [8] Borane and alane reductions of bulky N,N′-diaryl-1,3-diimines: structural characterization of products and intermediates in the diastereoselective synthesis of 1,3-diamines†
- [9] Hybrid multiple standard additions-analyte addition method for ion-selective electrodes with integral calibration
- [10] Three-dimensional crimped biodegradable poly(lactic acid) fibers prepared via melt spinning and controlled structural reorganization

Journal Name:New Journal of Chemistry
Research Products
-
CAS no.: 1076-07-9
-
CAS no.: 178064-02-3