Unsupervised deep learning for molecular dynamics simulations: a novel analysis of protein–ligand interactions in SARS-CoV-2 Mpro†
RSC Advances Pub Date: 2023-11-22 DOI: 10.1039/D3RA06375E
Abstract
Molecular dynamics (MD) simulations, which are central to drug discovery, offer detailed insights into protein–ligand interactions. However, analyzing large MD datasets remains a challenge. Current machine-learning solutions are predominantly supervised and have data labelling and standardisation issues. In this study, we adopted an unsupervised deep-learning framework, previously benchmarked for rigid proteins, to study the more flexible SARS-CoV-2 main protease (Mpro). We ran MD simulations of Mpro with various ligands and refined the data by focusing on binding-site residues and time frames in stable protein conformations. The optimal descriptor chosen was the distance between the residues and the center of the binding pocket. Using this approach, a local dynamic ensemble was generated and fed into our neural network to compute Wasserstein distances across system pairs, revealing ligand-induced conformational differences in Mpro. Dimensionality reduction yielded an embedding map that correlated ligand-induced dynamics and binding affinity. Notably, the high-affinity compounds showed pronounced effects on the protein's conformations. We also identified the key residues that contributed to these differences. Our findings emphasize the potential of combining unsupervised deep learning with MD simulations to extract valuable information and accelerate drug discovery.
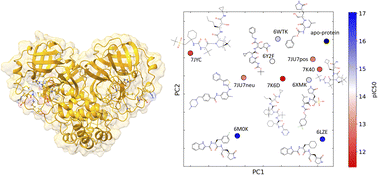
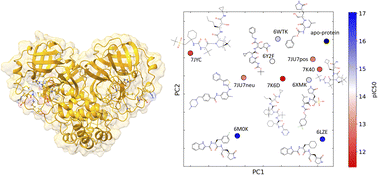
Recommended Literature
- [1] Ultrafast channel I and channel II charge generation processes at a nonfullerene donor–acceptor PTB7:PDI interface is crucial for its excellent photovoltaic performance†
- [2] Back cover
- [3] Hydrogen bond-free flavin redox properties: managing flavins in extreme aprotic solvents†
- [4] Characterizing the assembly behaviors of human amylin: a perspective derived from C-terminal variants†
- [5] Polyurethane with an ionic liquid crosslinker: a new class of super shape memory-like polymers†
- [6] Enzyme-responsive copper sulphidenanoparticles for combined photoacoustic imaging, tumor-selective chemotherapy and photothermal therapy†
- [7] Transfer hydrogenation of phenol over Co-CoOx/N-doped carbon: boosted catalyst performance enabled by synergistic catalysis between Co0 and Coδ+†
- [8] Back cover
- [9] Back cover
- [10] Impact of compatible solutes on the mechanical properties of fibronectin: a single molecule analysis

Journal Name:RSC Advances
Research Products
-
CAS no.: 16187-03-4
-
CAS no.: 1517-51-7
-
CAS no.: 131159-39-2
-
7-Fluoro-2-methylquinolin-4-ol
CAS no.: 18529-03-8